Scott Tominaga Explores the Aspects of Algorithmic and Quantitative Investing
3 min read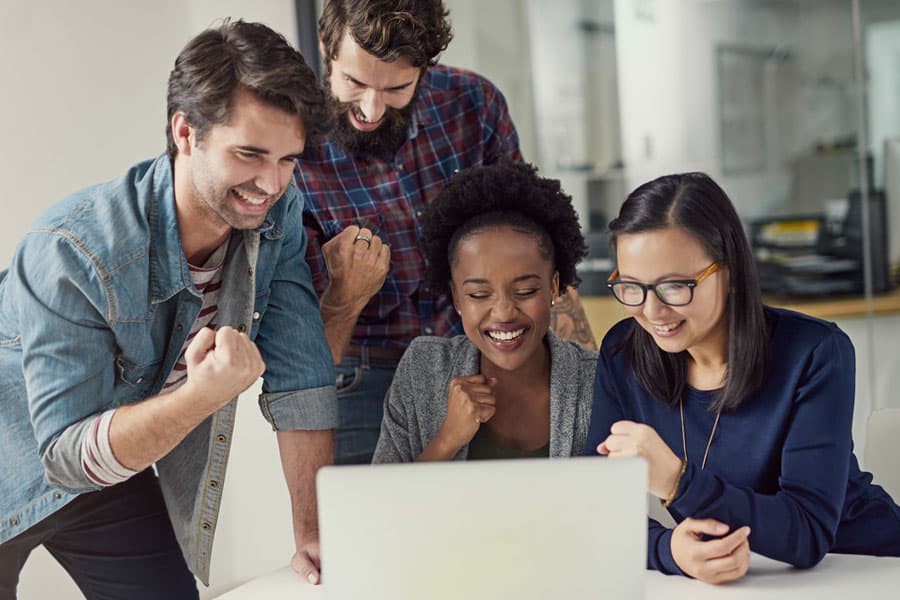
According to Scott Tominaga, algorithmic and quantitative investing leverages mathematical models, statistical analysis, and computational power to make data-driven investment decisions. By removing emotional bias and enhancing precision, these methods have become integral to modern financial markets. Below are key techniques that define this approach, offering insights into how institutions and investors optimize returns while managing risk.
Statistical Arbitrage
Statistical arbitrage identifies pricing inefficiencies between related securities. Using historical data, algorithms detect patterns or divergences from mean values. These strategies often exploit short-term mispricing in pairs of stocks, futures, or ETFs. Mean reversion principles guide trades, assuming prices will eventually converge. Execution relies on high-frequency data and rapid order placement to capitalize on fleeting opportunities.
Machine Learning Models
Machine learning algorithms analyse vast datasets to predict asset price movements. Supervised learning techniques, like regression and neural networks, forecast trends based on labeled training data. Unsupervised learning uncovers hidden patterns in unstructured data, such as news sentiment or trading volumes. Reinforcement learning adapts strategies dynamically by rewarding profitable decisions. These models continuously evolve, improving accuracy as new data becomes available.
Portfolio Optimization
Modern Portfolio Theory (MPT) underpins quantitative portfolio construction as per Scott Tominaga. Algorithms balance risk and return by diversifying assets with low correlation. Mean-variance optimization selects weightings that maximize expected returns for a given risk tolerance. Advanced methods, such as Black-Litterman models, incorporate investor views and market equilibrium assumptions. Real-time re-balancing ensures portfolios align with evolving market conditions.
High-Frequency Trading (HFT)
HFT executes orders in milliseconds, exploiting microsecond price discrepancies. Strategies include market-making, latency arbitrage, and order flow anticipation. Firms invest heavily in infrastructure—low-latency networks, co-located servers—to gain speed advantages. While controversial, HFT provides liquidity and narrows bid-ask spreads, benefiting broader markets.
Sentiment Analysis
Natural language processing (NLP) algorithms parse news articles, social media, and earnings calls to gauge market sentiment. Sentiment scores quantify bullish or bearish trends, influencing trading signals. Real-time analysis allows swift reactions to geopolitical events or corporate announcements. This technique bridges qualitative information with quantitative decision-making.
Risk Management Frameworks
Quantitative risk models assess potential losses using Value at Risk (VaR), stress testing, and scenario analysis. Monte Carlo simulations generate probabilistic outcomes under varying market conditions. Algorithms automatically adjust positions or hedge exposures using derivatives. Robust risk management ensures strategies remain viable during volatility or black swan events.
Backtesting and Validation
Historical back-testing evaluates strategy performance using past data. Metrics like Sharpe ratio, maximum drawdown, and win rate validate effectiveness. Walk-forward testing splits data into training and testing periods to avoid over-fitting. Out-of-sample testing confirms models generalize well to unseen data. Rigorous validation minimizes the risk of deploying flawed strategies.
Factor Investing
Factor-based strategies target specific return drivers, such as value, momentum, or quality. Algorithms rank securities based on predefined factors and construct portfolios over-weighting top performers. Multi-factor models combine several drivers to enhance diversification. This approach systematizes traditional investment styles, reducing reliance on discretionary judgment.
Market Microstructure Analysis
This technique studies how market mechanics—order types, liquidity, and transaction costs—impact execution. Algorithms optimize trade timing, size, and routing to minimize slippage. Understanding microstructure helps design strategies compatible with exchange rules and liquidity constraints, particularly in fragmented markets.
Execution Algorithms
Smart order routing splits large orders to avoid market impact. Implementation shortfall algorithms balance urgency with cost, using benchmarks like VWAP or TWAP. Dark pool matching conceals order intentions to prevent price movements. These algorithms ensure efficient trade execution while preserving alpha.
The integration of these techniques underscores the sophistication of algorithmic and quantitative investing. By combining statistical rigor, computational speed, and adaptive learning, investors navigate complex markets with heightened efficiency. According to Scott Tominaga, as technology advances, these methods will evolve, further blurring the line between human intuition and machine precision. Success in this field demands continuous innovation, disciplined risk management, and an unwavering commitment to data-driven decision-making.